stats homework using R
Homework- lab 5:
#The central limit theorem (CLT) states that as the sample size gets sufficiently large, the distribution of the sample means will be normally distributed.
#In addition, the CLT has been used to justify the fact that for many of our statistics we rely upon computing the mean (not median or trimmed mean) of our samples
#There are a few problems with the CLT.
#1) How large of a sample is needed
#2) It seems that our experiments with the contaminated normal may contradict this.
#In this homework assignment you will investigate the CLT further.
#PART 1 – The Central Limit Theorem under Normality.
#1.1) Simulate a standard normal population of 1 million people called pop1
#1.2) Draw 5000 samples of size 20 and put these in sam20. Draw 5000 samples of size 50 and put these in sam50 .
#1.3) Create variables called sam20means and sam50means that contains the means of the samples . Use a density plot to show the sampling distribution of the means for sam20means and sam50means together
#1.4) Compare the Standard Error (SE) of the sampling distributions. Which sample size creates better estimates of the population mean (ie. has the lowest SE)?
#PART 2 – The Central Limit Theorem under Non-Normality
#2.1) Simulate a contaminated normal population using cnorm() of 1 million people called pop2 where 30% (epsilon=0.3) of the data have an SD of 30 (k=30) .
#2.2) Draw 5000 samples of size 30 and put these in sam30. Draw 5000 samples of size 100 and put these in sam100.
#2.3) Create variables called sam30means, sam30tmeans, sam100means, sam100tmeans that represent the means AND trimmed means for the samples.
#2.4) Use a density plot to show the sampling distribution of the means and trimmed means for these variables.
#2.5) Compare the Standard Error (SE) of the sampling distributions.
#2.6) Which would be better here: a larger sample size using the mean as the location estimator OR a smaller sample using the trimmed mean?
#2.7) Which location estimator performs the best, regardless of sample size?
————————————————————————————————————————————————-
Lab 5 lecture notes:
#Lab 5-Contents
# 1. Sampling Distribution of the Mean,
# Median, and Trimmed Mean under Normality
# 2. Sampling Distribution of the Mean,
# Median, and Trimmed Mean under Non-Normality
# 3. The Central Limit Theorem
# Last week we saw that when we had a Normal or Uniform population,
# that the means of random samples taken from that population
#were normally distributed.
#Today we are going to investigate the distributions of the mean,
#median, and trimmed mean from samples coming from Normal
# and non-normal populations.
#———————————————————————————
# 1. Sampling Distribution of the Mean, Median,
# and Trimmed Mean under Normality
#———————————————————————————
#Let’s start by generating a standard normal distribution (mean=0, SD=1) for 1 million subjects
pop1 = rnorm(1000000, mean=0, sd=1)
#We will use this as our population from a normal distribution
#*#*#*#*#*#*#*#*#*#*#*#*#*#*#*#*#*#*#*#*#*#*#*#*#*#*#*#*#*#*#*#*#*#*#*#*#*#*#*#*#*#*#*#*#*#*#*#*#
#EXERCISE 1-1:
#A) Find the mean, median, trimmed mean (using tmean() ), and sd of pop1
#B) Draw a density plot of pop1
#*#*#*#*#*#*#*#*#*#*#*#*#*#*#*#*#*#*#*#*#*#*#*#*#*#*#*#*#*#*#*#*#*#*#*#*#*#*#*#*#*#*#*#*#*#*#*#*#
#A)
mean(pop1); median(pop1);
tmean(pop1); sd(pop1)
#B)
plot(density(pop1))
#Like we did last week, we are going to want to take random samples
# from our population and then compute a measure of central tendency
#(eg. mean, median, trimmed mean) for each sample and examine
#the distribution of this measure.
#We are going to take 5000 samples of 20 subjects
sam1 = matrix(, ncol=5000, nrow=20)
#*#*#*#*#*#*#*#*#*#*#*#*#*#*#*#*#*#*#*#*#*#*#*#*#*#*#*#*#*#*#*#*#*#*#*#*#*#*#*#*#*#*#*#*#*#*#*#*#
# EXERCISE 1-2: Use a loop to draw 5000 samples of size 20 from pop1
# an place the samples in sam1
#*#*#*#*#*#*#*#*#*#*#*#*#*#*#*#*#*#*#*#*#*#*#*#*#*#*#*#*#*#*#*#*#*#*#*#*#*#*#*#*#*#*#*#*#*#*#*#*#
for (ii in 1:5000) {
sam1[ , ii] = sample(pop1, 20, replace=TRUE)
}
# Now that we have our datafile containing all 5000 samples (ie. sam1)
# we can begin to create variables for each of our location measures
#I’ll start us off with the mean
sam1means = apply(sam1, 2, mean) # number 2 = work in the columns rather than rows
#*#*#*#*#*#*#*#*#*#*#*#*#*#*#*#*#*#*#*#*#*#*#*#*#*#*#*#*#*#*#*#*#*#*#*#*#*#*#*#*#*#*#*#*#*#*#*#*#*#*#*#*#*#*#*#*#
# EXERCISE 1-3: Use the apply function to generate
# the variables sam1meds (medians) and sam1tmeans (trimmed mean)
#*#*#*#*#*#*#*#*#*#*#*#*#*#*#*#*#*#*#*#*#*#*#*#*#*#*#*#*#*#*#*#*#*#*#*#*#*#*#*#*#*#*#*#*#*#*#*#*#*#*#*#*#*#*#*#*#
sam1meds = apply(sam1, 2, median)
sam1tmeans = apply(sam1, 2, tmean)
#Let’s look at the distributions of each of these location estimators
plot(density(sam1means))
lines(density(sam1meds), col=”red”)
lines(density(sam1tmeans), col=”blue”)
abline(v = mean(pop1), lty=2) #Add in a line for the pop1 mean
#??????????????????????????????????????????????????????????????#
#Thought Question 1: Which location estimator performs the best
#for data coming from a normal population? Why?
#??????????????????????????????????????????????????????????????#
# One of the ways we can determine which location estimator
# performs the best is by looking at the standard deviation
# of the estimator accross all the samples.
# The estimator with the lowest SD will have the least amount
# of variability accross the samples.
# A more common name for the standard deviation of the location
# estimator is called the Standard Error or SE
#*#*#*#*#*#*#*#*#*#*#*#*#*#*#*#*#*#*#*#*#*#*#*#*#*#*#*#*#*#*#*#*#*#*#*#*#*#*#*#*#*#*#*#*#*#*#*#*#*#*#*#*#*#*#*#*#
# EXERCISE 1-4: Find the Standard Error of the sample means,
# medians, and trimmed means. Based upon the SE, which
# location estimator is the best for samples coming from
# a normal population?
#*#*#*#*#*#*#*#*#*#*#*#*#*#*#*#*#*#*#*#*#*#*#*#*#*#*#*#*#*#*#*#*#*#*#*#*#*#*#*#*#*#*#*#*#*#*#*#*#*#*#*#*#*#*#*#*#
sd(sam1means); sd(sam1meds); sd(sam1tmeans)
#The mean performs the best.
# In real life, we generally cannot go out an collect multiple samples
# from a population, so we compute the Standard Error using a formula:
# SE = sd(sample) / sqrt(sample N)
#———————————————————————————
# 2. Sampling Distribution of the Mean, Median,
# and Trimmed Mean under Non-Normality
#———————————————————————————
# Normal distributions generally have very few outliers,
# however when outliers begin to occur more frequently so of the
# basic assumptions about normal distributions are no longer true
# (as we are about to see).
# One distribution that is like a normal distribution,
# but with more outliers is called a mixed or contaminated
# normal distribution and it is a result of two populations mixing together.
#*#*#*#*#*#*#*#*#*#*#*#*#*#*#*#*#*#*#*#*#*#*#*#*#*#*#*#*#*#*#*#*#*#*#*#*#*#*#*#*#*#*#*#*#*#*#*#*#
#EXAMPLE 1: “a” will be a mix of TWO populations 1: with SD=1 and 2: with SD=2
a=c(rnorm(5000, 0, 1), rnorm(5000, 0, 2))
#Let’s compare this to b, which is from ONE population but with the same parameters of a
b=rnorm(10000, mean(a), sd(a))
plot(density(a))
lines(density(b), col=”red”)
#*#*#*#*#*#*#*#*#*#*#*#*#*#*#*#*#*#*#*#*#*#*#*#*#*#*#*#*#*#*#*#*#*#*#*#*#*#*#*#*#*#*#*#*#*#*#*#*#
#??????????????????????????????????????????????????????????????#
#Thought Question 2: How are a and b from Example 1 different?
#??????????????????????????????????????????????????????????????#
#Thankfully, rather than having to create contaminated normal distributions the hard way, we can just use
#a function provided to us by Dr. Wilcox called cnorm()
#^^^^^^^^^^^^^^^^^^^^^^^^^^^^^^^^^^^^^^^^^^^^^^^^^^^^^^^^^^^^^^^^#
#Contaminated/Mix Normal Distribution: cnorm(n, epsilon=0.1, k=10)
#^^^^^^^^^^^^^^^^^^^^^^^^^^^^^^^^^^^^^^^^^^^^^^^^^^^^^^^^^^^^^^^^^#
#Let’s look at the options for the contaminated normal distribution:
#cnorm() combines two normal distributions:
#1) A standard normal (mean=0, sd=1) for 1-epsilon % of the data
#2) A normal of mean=0 and sd=k for epsilon % of the data
#If we were trying to re-create the variable a we made in example 1 we would have to do:
z=cnorm(10000, epsilon=0.5, k=2)
plot(density(a))
lines(density(z), col=”blue”)
#Which looks very very similar to a!
#Let’s create a second population called pop2 from a contaminated normal distribution
pop2 = cnorm(1000000, epsilon=0.1, k=10)
#The mean, sd, and plot of which are:
mean(pop2); sd(pop2); plot(density(pop2))
#*#*#*#*#*#*#*#*#*#*#*#*#*#*#*#*#*#*#*#*#*#*#*#*#*#*#*#*#*#*#*#*#*#*#*#*#*#*#*#*#*#*#*#*#*#*#*#*#*#*#*#*#*#*#*#*#
#EXERCISE 2:
#A) Create an empty matrix called sam2 to contain 5000 samples
#of 20 observations each
#B) Populate sam2 with 5000 random samples of size 20 from pop2
#C) Compute the mean (sam2means), median (sam2meds),
#and trimmed mean (sam2tmeans) for each sample
#D) Create an overlaid density plot of each sample WITH the pop2
#mean as a verticle line
#E) Find the SE of each location estimator
#F) Based upon the SE, which location estimator is the best
# for samples coming from a contaminated normal distribution
#*#*#*#*#*#*#*#*#*#*#*#*#*#*#*#*#*#*#*#*#*#*#*#*#*#*#*#*#*#*#*#*#*#*#*#*#*#*#*#*#*#*#*#*#*#*#*#*#*#*#*#*#*#*#*#*#
#A)
#B)
#C)
#D)
#E)
#F)
#———————————————————————————
# 3. The Central Limit Theorem
#———————————————————————————
#We’ve discovered a few things today:
#1) When a population comes from a normal distribution,
# then mean will be the best location estimator of the samples
#2) When a population comes from a mixed/contaminated normal distribution,
# the trimmed mean is the best location estimator
# These observations are related to the Central Limit Theorem (CLT)
# that is discussed in Section 5.3 of the book (page 85)
# The CLT states that as the sample size gets sufficiently large,
# the distribution of the sample means will be normally distributed.
# We saw a demonstration of this last week when we looked at the means
# from the unifom distribution.
# The CLT has been used to justify the fact that for many of our statistics
# we rely upon computing the mean (not median or trimmed mean) of our samples
#There are a few problems with the CLT.
#1) how large of a sample do we need?
#2) It seems that our experiements with the contaminated normal may contradict this.
#In the homework you will investigate this further
Looking for a solution written from scratch with No plagiarism and No AI?
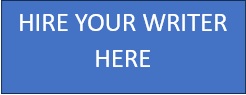
WHY CHOOSE US?
We deliver quality original papers |
Our experts write quality original papers using academic databases.We dont use AI in our work. We refund your money if AI is detected |
Free revisions |
We offer our clients multiple free revisions just to ensure you get what you want. |
Discounted prices |
All our prices are discounted which makes it affordable to you. Use code FIRST15 to get your discount |
100% originality |
We deliver papers that are written from scratch to deliver 100% originality. Our papers are free from plagiarism and NO similarity.We have ZERO TOLERANCE TO USE OF AI |
On-time delivery |
We will deliver your paper on time even on short notice or short deadline, overnight essay or even an urgent essay |